Understanding the concept of Casper Undefined Quartile is essential for anyone navigating the complexities of modern financial analysis and data interpretation. As the financial world evolves, understanding terms like "Casper Undefined Quartile" becomes increasingly relevant for investors, analysts, and enthusiasts alike. This term, while seemingly complex, holds valuable insights into how data is categorized and interpreted in various contexts.
The term "Casper Undefined Quartile" might initially sound obscure, but it has significant implications in statistical analysis and financial modeling. Quartiles, as a statistical tool, are used to divide datasets into four equal parts, helping analysts understand the distribution of data. The "undefined" aspect arises when data points fall outside expected parameters, creating unique challenges and opportunities for interpretation.
As we delve deeper into this topic, we will explore the origins of the term, its applications in various fields, and how it can impact decision-making processes. Whether you're a seasoned professional or a curious beginner, this article aims to demystify the concept and provide actionable insights for your analytical toolkit.
Read also:Exploring Carla Cute Vr A Comprehensive Guide To The Phenomenon
Table of Contents
- Introduction to Casper Undefined Quartile
- Defining Casper Undefined Quartile
- Historical Context of Quartiles
- Applications in Financial Analysis
- Data Interpretation Challenges
- Statistical Methods for Quartile Analysis
- Case Studies: Real-World Examples
- Software Tools for Analysis
- Future Trends in Quartile Analysis
- Conclusion and Call to Action
Introduction to Casper Undefined Quartile
In the realm of data analysis, the term "Casper Undefined Quartile" refers to a specific scenario where traditional quartile calculations encounter anomalies. These anomalies often arise due to incomplete datasets, outliers, or unconventional data distributions. Understanding these scenarios is crucial for analysts aiming to make informed decisions based on statistical data.
Why Quartiles Matter
Quartiles play a pivotal role in dividing datasets into manageable segments, allowing analysts to identify trends, patterns, and potential outliers. The "undefined" aspect in Casper Undefined Quartile highlights the challenges faced when data does not conform to expected distributions, making it a critical area of study for statisticians and analysts alike.
Common Misconceptions
Many beginners assume that all datasets can be neatly divided into quartiles without complications. However, real-world data often presents challenges such as missing values or extreme outliers, leading to the "undefined" quartile scenario. Addressing these issues requires a deeper understanding of statistical methods and tools.
Defining Casper Undefined Quartile
The term "Casper Undefined Quartile" specifically refers to a situation where the standard calculation of quartiles becomes ambiguous due to data irregularities. This can occur when data points are missing, when datasets are too small to provide meaningful divisions, or when extreme values skew the distribution.
Key Characteristics
- Data irregularities leading to ambiguous quartile boundaries.
- Small sample sizes that hinder accurate division into quartiles.
- Outliers significantly affecting the distribution of data.
Historical Context of Quartiles
The concept of quartiles dates back to the early days of statistical analysis, where they were first used to categorize and analyze datasets. Over time, the methodology has evolved, but the core principle of dividing data into four equal parts remains unchanged. Understanding the historical context of quartiles helps in appreciating the challenges posed by the "undefined" scenario.
Evolution of Quartile Analysis
From manual calculations to advanced software tools, the evolution of quartile analysis reflects the broader advancements in data science. Modern tools have made it easier to handle large datasets, but the challenge of dealing with "undefined" scenarios persists, requiring innovative solutions.
Read also:Unleash Your Inner Jackie Brown The Ultimate Guide To Jackie Brown Costumes
Applications in Financial Analysis
In the financial sector, quartile analysis is widely used to evaluate investment portfolios, assess risk, and identify trends. The "Casper Undefined Quartile" scenario can have significant implications for financial analysts, as it may lead to inaccurate conclusions if not properly addressed.
Risk Assessment
When dealing with undefined quartiles, financial analysts must exercise caution to ensure that risk assessments remain accurate. This involves employing advanced statistical techniques and leveraging robust software tools to handle data irregularities effectively.
Data Interpretation Challenges
Interpreting data in the context of Casper Undefined Quartile requires a nuanced understanding of statistical principles. Analysts must be adept at identifying and addressing anomalies to ensure that their conclusions are valid and actionable.
Common Challenges
- Identifying and handling missing data points.
- Addressing the impact of outliers on quartile calculations.
- Ensuring data accuracy and consistency across datasets.
Statistical Methods for Quartile Analysis
Several statistical methods can be employed to address the challenges posed by Casper Undefined Quartile. These methods include interpolation, data imputation, and robust statistical techniques designed to handle irregularities in data distribution.
Interpolation Techniques
Interpolation is a powerful tool for estimating missing data points, allowing analysts to calculate quartiles more accurately. By bridging gaps in datasets, interpolation helps mitigate the effects of undefined quartiles.
Case Studies: Real-World Examples
Examining real-world case studies provides valuable insights into how Casper Undefined Quartile scenarios are handled in practice. These examples illustrate the challenges faced by analysts and the solutions they employ to overcome them.
Case Study 1: Financial Portfolio Analysis
In a case involving a financial portfolio, analysts encountered an undefined quartile due to missing data points. By employing data imputation techniques, they were able to accurately assess the portfolio's performance and make informed investment decisions.
Case Study 2: Market Trend Analysis
A market trend analysis faced challenges due to extreme outliers affecting quartile calculations. The analysts used robust statistical methods to address these issues, ensuring that their conclusions remained valid and actionable.
Software Tools for Analysis
Modern software tools have revolutionized the way analysts handle complex datasets and address Casper Undefined Quartile scenarios. These tools provide advanced features for data cleaning, interpolation, and statistical analysis, empowering analysts to make more accurate decisions.
Popular Tools
- Excel: Ideal for basic data analysis and visualization.
- R: A powerful programming language for advanced statistical analysis.
- Python: Offers extensive libraries for data manipulation and analysis.
Future Trends in Quartile Analysis
As data science continues to evolve, new trends in quartile analysis are emerging. These trends include the integration of artificial intelligence and machine learning techniques to handle complex datasets and address undefined scenarios more effectively.
AI and Machine Learning
AI-driven tools are increasingly being used to automate data cleaning and interpolation processes, reducing the burden on analysts and improving the accuracy of quartile calculations. These advancements promise to enhance the reliability of data analysis in the future.
Conclusion and Call to Action
In conclusion, understanding Casper Undefined Quartile is essential for anyone involved in data analysis, particularly in the financial sector. By addressing the challenges posed by undefined scenarios and leveraging advanced statistical methods and tools, analysts can make more informed and accurate decisions.
We invite you to share your thoughts and experiences in the comments section below. Additionally, explore our other articles for more insights into data analysis and financial modeling. Together, we can continue to expand our knowledge and improve our analytical capabilities.
Data sources and references: Statista, Investopedia, World Bank.

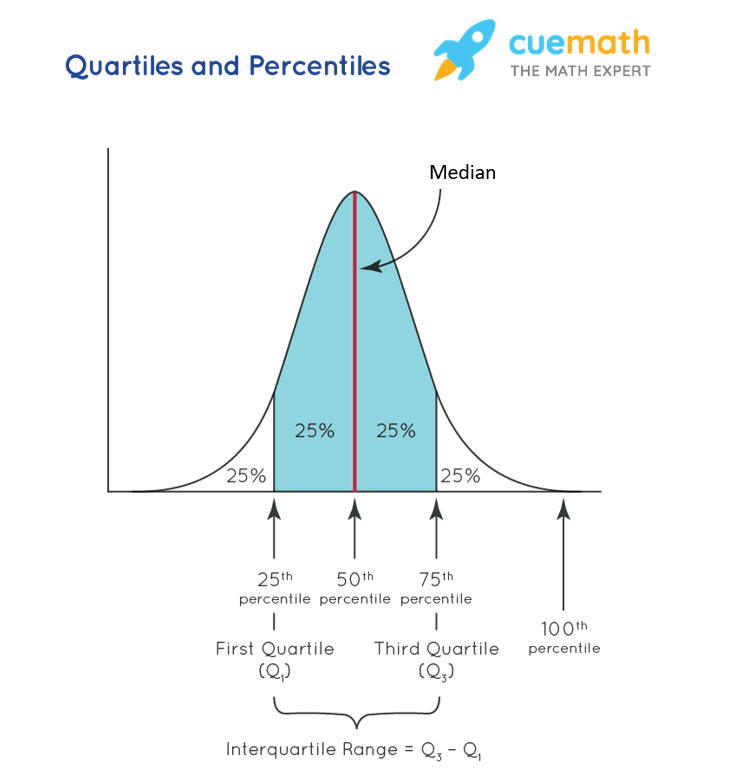
